WHEN TAY MADE HER DEBUT in March 2016, Microsoft had high hopes for the artificial intelligence-powered "social chatbot." Like the automated, text-based chat programs that many people had already encountered on e-commerce sites and in customer service conversations, Tay could answer written questions; by doing so on Twitter and other social media, she could engage with the masses.
But rather than simply doling out facts, Tay was engineered to converse in a more sophisticated way--one that had an emotional dimension. She would be able to show a sense of humor, to banter with people like a friend. Her creators had even engineered her to talk like a wisecracking teenage girl. When Twitter users asked Tay who her parents were, she might respond, "Oh a team of scientists in a Microsoft lab. They're what u would call my parents." If someone asked her how her day had been, she could quip, "omg totes exhausted."
Best of all, Tay was supposed to get better at speaking and responding as more people engaged with her. As her promotional material said, "The more you chat with Tay the smarter she gets, so the experience can be more personalized for you." In low-stakes form, Tay was supposed to exhibit one of the most important features of true A.I.--the ability to get smarter, more effective, and more helpful over time.
But nobody predicted the attack of the trolls.
Realizing that Tay would learn and mimic speech from the people she engaged with, malicious pranksters across the web deluged her Twitter feed with racist, homophobic, and otherwise offensive comments. Within hours, Tay began spitting out her own vile lines on Twitter, in full public view. "Ricky gervais learned totalitarianism from adolf hitler, the inventor of atheism," Tay said, in one tweet that convincingly imitated the defamatory, fake-news spirit of Twitter at its worst. Quiz her about then-president Obama, and she'd compare him to a monkey. Ask her about the Holocaust, and she'd deny it occurred.
In less than a day, Tay's rhetoric went from family-friendly to foulmouthed; fewer than 24 hours after her debut, Microsoft took her offline and apologized for the public debacle.
What was just as striking was that the wrong turn caught Microsoft's research arm off guard. "When the system went out there, we didn't plan for how it was going to perform in the open world," Microsoft's managing director of research and artificial intelligence, Eric Horvitz, told Fortune in a recent interview.
http://fortunedotcom.files.wordpress.com/2018/06/rac-07-18-sidebar.png?w=150&h=91 150w, http://fortunedotcom.files.wordpress.com/2018/06/rac-07-18-sidebar.png?w=300&h=183 300w, http://fortunedotcom.files.wordpress.com/2018/06/rac-07-18-sidebar.png?w=768&h=467 768w" sizes="(max-width: 1024px) 100vw, 1024px">
After Tay's meltdown, Horvitz immediately asked his senior team working on "natural language processing"--the function central to Tay's conversations--to figure out what went wrong. The staff quickly determined that basic best practices related to chatbots were overlooked. In programs that were more rudimentary than Tay, there were usually protocols that blacklisted offensive words, but there were no safeguards to limit the type of data Tay would absorb and build on.
Today, Horvitz contends, he can "love the example" of Tay--a humbling moment that Microsoft could learn from. Microsoft now deploys far more sophisticated social chatbots around the world, including Ruuh in India, and Rinna in Japan and Indonesia. In the U.S., Tay has been succeeded by a social-bot sister, Zo. Some are now voice-based, the way Apple's Siri or Amazon's Alexa are. In China, a chatbot called Xiaoice is already "hosting" TV shows and sending chatty shopping tips to convenience store customers.
Still, the company is treading carefully. It rolls the bots out slowly, Horvitz explains, and closely monitors how they are behaving withthe p ublic as they scale. But it's sobering to realize that, even though A.I. tech has improved exponentially in the intervening two years, the work of policing the bots' behavior never ends. The company's staff constantly monitors the dialogue for any changes in its behavior. And those changes keep coming. In its early months, for example, Zo had to be tweaked and tweaked again after separate incidents in which it referred to Microsoft's flagship Windows software as "spyware" and called the Koran, Islam's foundational text, "very violent."
Can This Startup Break Big Tech's Hold on A.I.?
To be sure, Tay and Zo are not our future robot overlords. They're relatively primitive programs occupying the parlor-trick end of the research spectrum, cartoon shadows of what A.I. can accomplish. But their flaws highlight both the power and the potential pitfalls of software imbued with even a sliver of artificial intelligence. And they exemplify more insidious dangers that are keeping technologists awake at night, even as the business world prepares to entrust ever more of its future to this revolutionary new technology.
"You get your best practices in place, and hopefully those things will get more and more rare," Horvitz says. With A.I. rising to the top of every company's tech wish list, figuring out those practices has never been more urgent.
http://fortunedotcom.files.wordpress.com/2018/06/f1.png?w=150&h=15 150w, http://fortunedotcom.files.wordpress.com/2018/06/f1.png?w=300&h=29 300w, http://fortunedotcom.files.wordpress.com/2018/06/f1.png?w=768&h=75 768w" sizes="(max-width: 1024px) 100vw, 1024px">
FEW DISPUTE that we're on the verge of a corporate A.I. gold rush. By 2021, research firm IDC predicts, organizations will spend $52.2 billion annually on A.I.-related products--and economists and analysts believe they'll realize many billions more in savings and gains from that investment. Some of that bounty will come from the reduction in human headcount, but far more will come from enormous efficiencies in matching product to customer, drug to patient, solution to problem. Consultancy PwC estimates that A.I. could contribute up to $15.7 trillion to the global economy in 2030, more than the combined output of China and India today.
The A.I. renaissance has been driven in part by advances in "deep-learning" technology. With deep learning, companies feed their computer networks enormous amounts of information so that they recognize patterns more quickly, and with less coaching (and eventually, perhaps, no coaching) from humans. Facebook, Google, Microsoft, Amazon, and IBM are among the giants already using deep-learning tech in their products. Apple's Siri and Google Assistant, for example, recognize and respond to your voice because of deep learning. Amazon uses deep learning to help it visually screen tons of produce that it delivers via its grocery service.
And in the near future, companies of every size hope to use deep-learning-powered software to mine their data and find gems buried too deep for meager human eyes to spot. They envision A.I.-driven systems that can scan thousands of radiology images to more quickly detect illnesses, or screen multitudes of r?sum?s to save time for beleaguered human resources staff. In a technologist's utopia, businesses could use A.I. to sift through years of data to better predict their next big sale, a pharmaceutical giant could cut down the time it takes to discover a blockbuster drug, or auto insurers could scan terabytes of car accidents and automate claims.
But for all their enormous potential, A.I.-powered systems have a dark side. Their decisions are only as good as the data that humans feed them. As their builders are learning, the data used to train deep-learning systems isn't neutral. It can easily reflect the biases--conscious and unconscious--of the people who assemble it. And sometimes data can be slanted by history, encoding trends and patterns that reflect centuries-old discrimination. A sophisticated algorithm can scan a historical database and conclude that white men are the most likely to succeed as CEOs; it can't be programmed (yet) to recognize that, until very recently, people who weren't white men seldom got the chance to be CEOs. Blindness to bias is a fundamental flaw in this technology, and while executives and engineers speak about it only in the most careful and diplomatic terms, there's no doubt it's high on their agenda.
The most powerful algorithms being used today "haven't been optimized for any definition of fairness," says Deirdre Mulligan, an associate professor at the University of California at Berkeley who studies ethics in technology. "They have been optimized to do a task." A.I. converts data into decisions with unprecedented speed--but what scientists and ethicists are learning, Mulligan says, is that in many cases "the data isn't fair."
Adding to the conundrum is that deep learning is much more complex than the conventional algorithms that are its predecessors--making it trickier for even the most sophisticated programmers to understand exactly how an A.I. system makes any given choice. Like Tay, A.I. products can morph to behave in ways that its creators don't intend and can't anticipate. And because the creators and users of these systems religiously guard the privacy of their data and algorithms, citing competitive concerns about proprietary technology, it's hard for external watchdogs to determine what problems could be embedded in any given system.
The fact that tech that includes these black-box mysteries is being productized and pitched to companies and governments has more than a few researchers and activists deeply concerned. "These systems are not just off-the-shelf software that you can buy and say, 'Oh, now I can do accounting at home,' " says Kate Crawford, principal researcher at Microsoft and codirector of the AI Now Institute at New York University. "These are very advanced systems that are going to be influencing our core social institutions."
http://fortunedotcom.files.wordpress.com/2018/06/t2.png?w=150&h=15 150w, http://fortunedotcom.files.wordpress.com/2018/06/t2.png?w=300&h=29 300w, http://fortunedotcom.files.wordpress.com/2018/06/t2.png?w=768&h=75 768w" sizes="(max-width: 1024px) 100vw, 1024px">
THOUGH THEY MAY not think of it as such, most people are familiar with at least one A.I. breakdown: the spread of fake news on Facebook's ubiquitous News Feed in the run-up to the 2016 U.S. presidential election.
The social media giant and its data scientists didn't create flat-out false stories. But the algorithms powering the News Feed weren't designed to filter "false" from "true"; they were intended to promote content personalized to a user's individual taste. While the company doesn't disclose much about its algorithms (again, they're proprietary), it has acknowledged that the calculus involves identifying stories that other users of similar tastes are reading and sharing. The result: Thanks to an endless series of what were essentially popularity contests, millions of people's personal News Feeds were populated with fake news primarily because their peers liked it.
While Facebook offers an example of how individual choices can interact toxically with A.I., researchers worry more about how deep learning could read, and misread, collective data. Timnit Gebru, a postdoctoral researcher who has studied the ethics of algorithms at Microsoft and elsewhere, says she's concerned about how deep learning might affect the insurance market--a place where the interaction of A.I. and data could put minority groups at a disadvantage. Imagine, for example, a data set about auto accident claims. The data shows that accidents are more likely to take place in inner cities, where densely packed populations create more opportunities for fender benders. Inner cities also tend to have disproportionately high numbers of minorities among their residents.
A deep-learning program, sifting through data in which these correlations were embedded, could "learn" that there was a relationship between belonging to a minority and having car accidents, and could build that lesson into its assumptions about all drivers of color. In essence, that insurance A.I. would develop a racial bias. And that bias could get stronger if, for example, the system were to be further "trained" by reviewing photos and video from accidents in inner-city neighborhoods. In theory, the A.I. would become more likely to conclude that a minority driver is at fault in a crash involving multiple drivers. And it's more likely to recommend charging a minority driver higher premiums, regardless of her record.
It should be noted that insurers say they do not discriminate or assign rates based on race. But the inner-city hypothetical shows how data that seems neutral (facts about where car accidents happen) can be absorbed and interpreted by an A.I. system in ways that create new disadvantages (algorithms that charge higher prices to minorities, regardless of where they live, based on their race).
What's more, Gebru notes, given the layers upon layers of data that go into a deep-learning system's decision-making, A.I.-enabled software could make decisions like this without engineers realizing how or why. "These are things we haven't even thought about, because we are just starting to uncover biases in the most rudimentary algorithms," she says.
What distinguishes modern A.I.-powered software from earlier generations is that today's systems "have the ability to make legally significant decisions on their own," says Matt Scherer, a labor and employment lawyer at Littler Mendelson who specializes in A.I. The idea of not having a human in the loop to make the call about key outcomes alarmed Scherer when he started studying the field. If flawed data leads a deep-learning-powered X-ray to miss an overweight man's tumor, is anyone responsible? "Is anyone looking at the legal implications of these things?" Scherer
asks himself.
http://fortunedotcom.files.wordpress.com/2018/06/a.png?w=150&h=15 150w, http://fortunedotcom.files.wordpress.com/2018/06/a.png?w=300&h=29 300w, http://fortunedotcom.files.wordpress.com/2018/06/a.png?w=768&h=75 768w" sizes="(max-width: 1024px) 100vw, 1024px">
AS BIG TECH PREPARES to embed deep-learning technology in commercial software for customers, questions like this are moving from the academic "what if?" realm to the front burner. In 2016, the year of the Tay misadventure, Microsoft created an internal group called Aether, which stands for AI and Ethics in Engineering and Research, chaired by Eric Horvitz. It's a cross-disciplinary group, drawing representatives from engineering, research, policy, and legal teams, and machine-learning bias is one of its top areas of discussion. "Does Microsoft have a viewpoint on whether, for example, face-recognition software should be applied in sensitive areas like criminal justice and policing?" Horvitz muses, describing some of the topics the group is discussing. "Is the A.I. technology good enough to be used in this area, or will the failure rates be high enough where there has to be a sensitive, deep consideration for the costs of the failures?
Joaquin Qui?onero Candela leads Facebook's Applied Machine Learning group, which is responsible for creating the company's A.I. technologies. Among many other functions, Facebook uses A.I. to weed spam out of people's News Feeds. It also uses the technology to help serve stories and posts tailored to their interests--putting Candela's team adjacent to the fake-news crisis. Candela calls A.I. "an accelerator of history," in that the technology is "allowing us to build amazing tools that augment our ability to make decisions." But as he acknowledges, "It is in decision-making that a lot of ethical questions come into play."
Facebook's struggles with its News Feed show how difficult it can be to address ethical questions once an A.I. system is already powering a product. Microsoft was able to tweak a relatively simple system like Tay by adding profanities or racial epithets to a blacklist of terms that its algorithm should ignore. But such an approach wouldn't work when trying to separate "false" from "true"--there are too many judgment calls involved. Facebook's efforts to bring in human moderators to vet news stories--by, say, excluding articles from sources that frequently published verifiable falsehoods--exposed the company to charges of censorship. Today, one of Facebook's proposed remedies is to simply show less news in the News Feed and instead highlight baby pictures and graduation photos--a winning-byretreating approach.
Therein lies the heart of the challenge: The dilemma for tech companies isn't so much a matter of tweaking an algorithm or hiring humans to babysit it; rather, it's about human nature itself. The real issue isn't technical or even managerial--it's philosophical. Deirdre Mulligan, the Berkeley ethics professor, notes that it's difficult for computer scientists to codify fairness into software, given that fairness can mean different things to different people. Mulligan also points out that society's conception of fairness can change over time. And when it comes to one widely shared ideal of fairness--namely, that everybody in a society ought to be represented in that society's decisions--historical data is particularly likely to be flawed and incomplete.
One of the Microsoft Aether group's thought experiments illustrates the conundrum. It involves A.I. tech that sifts through a big corpus of job applicants to pick out the perfect candidate for a top executive position. Programmers could instruct the A.I. software to scan the characteristics of a company's best performers. Depending on the company's history, it might well turn out that all of the best performers--and certainly all the highest ranking executives--were white males. This might overlook the possibility that the company had a history of promoting only white men (for generations, most companies did), or has a culture in which minorities or women feel unwelcome and leave before they rise.
Anyone who knows anything about corporate history would recognize these flaws--but most algorithms wouldn't. If A.I. were to automate job recommendations, Horvitz says, there's always a chance that it can "amplify biases in society that we may not be proud of."
FEI-FEI LI, the chief scientist for A.I. for Google's cloud-computing unit, says that bias in technology "is as old as human civilization"--and can be found in a lowly pair of scissors. "For centuries, scissors were designed by right-handed people, used by mostly right-handed people," she explains. "It took someone to recognize that bias and recognize the need to create scissors for lefthanded people." Only about 10% of the world's people are left-handed--and it's human nature for members of the dominant majority to be oblivious to the experiences of other groups.
That same dynamic, it turns out, is present in some of A.I.'s other most notable recent blunders. Consider the A.I.-powered beauty contest that Russian scientists conducted in 2016. Thousands of people worldwide submitted selfies for a contest in which computers would judge their beauty based on factors like the symmetry of their faces.
But of the 44 winners the machines chose, only one had dark skin. An international ruckus ensued, and the contest's operators later attributed the apparent bigotry of the computers on the fact that the data sets they used to train them did not contain many photos of people of color. The computers essentially ignored photos of people with dark skin and deemed those with lighter skin more "beautiful" because they represented the majority.
This bias-through-omission turns out to be particularly pervasive in deep-learning systems in which image recognition is a major part of the training process. Joy Buolamwini, a researcher at the MIT Media Lab, recently collaborated with Gebru, the Microsoft researcher, on a paper studying gender-recognition technologies from Microsoft, IBM, and China's Megvii. They found that the tech consistently made more accurate identifications of subjects with photos of lighter-skinned men than with those of darker-skinned women.
Such algorithmic gaps may seem trivial in an online beauty contest, but Gebru points out that such technology can be used in much more high-stakes situations. "Imagine a selfdriving car that doesn't recognize when it 'sees' black people," Gebru says. "That could have dire consequences."
The Gebru-Buolamwini paper is making waves. Both Microsoft and IBM have said they have taken actions to improve their image-recognition technologies in response to the audit. While those two companies declined to be specific about the steps they were taking, other companies that are tackling the problem offer a glimpse of what tech can do to mitigate bias.
When Amazon started deploying algorithms to weed out rotten fruit, it needed to work around a sampling-bias problem. Visual-recognition algorithms are typically trained to figure out what, say, strawberries are "supposed" to look like by studying a huge database of images. But pictures of rotten berries, as you might expect, are relatively rare compared with glamour shots of the good stuff. And unlike humans, whose brains tend to notice and react strongly to "outliers," machine-learning algorithms tend to discount or ignore them.
To adjust, explains Ralf Herbrich, Amazon's director of artificial intelligence, the online retail giant is testing a computer science technique called oversampling. Machine-learning engineers can direct how the algorithm learns by assigning heavier statistical "weights" to underrepresented data, in this case the pictures of the rotting fruit. The result is that the algorithm ends up being trained to pay more attention to spoiled food than that food's prevalence in the data library might suggest.
Herbrich points out that oversampling can be applied to algorithms that study humans too (though he declined to cite specific examples of how Amazon does so). "Age, gender, race, nationality--they are all dimensions that you specifically have to test the sampling biases for in order to inform the algorithm over time," Herbrich says. To make sure that an algorithm used to recognize faces in photos didn't discriminate against or ignore people of color, or older people, or overweight people, you could add weight to photos of such individuals to make up for the shortage in your data set.
The 9 Companies Behind the A.I. Acquisition Boom
Other engineers are focusing further "upstream"--making sure that the underlying data used to train algorithms is inclusive and free of bias, before it's even deployed. In image recognition, for example, the millions of images used to train deep-learning systems need to be examined and labeled before they are fed to computers. Radha Basu, the CEO of data-training startup iMerit, whose clients include Getty Images and eBay, explains that the company's staff of over 1,400 worldwide is trained to label photos on behalf of its customers in ways that can mitigate bias.
Basu declined to discuss how that might play out when labeling people, but she offered other analogies. iMerit staff in India may consider a curry dish to be "mild," while the company's staff in New Orleans may describe the same meal as "spicy." iMerit would make sure both terms appear in the label for a photo of that dish, because to label it as only one or the other would be to build an inaccuracy into the data. Assembling a data set about weddings, iMerit would include traditional Western white-dress-and-layer-cake images--but also shots from elaborate, more colorful weddings in India or Africa.
iMerit's staff stands out in a different way, Basu notes: It includes people with Ph.D.s, but also less-educated people who struggled with poverty, and 53% of the staff are women. The mix ensures that as many viewpoints as possible are involved in the data labeling process. "Good ethics does not just involve privacy and security," Basu says. "It's about bias, it's about, Are we missing a viewpoint?" Tracking down that viewpoint is becoming part of more tech companies' strategic agendas. Google, for example, announced in June that it would open an A.I. research center later this year in Accra, Ghana. "A.I. has great potential to positively impact the world, and more so if the world is well represented in the development of new A.I. technologies," two Google engineers wrote in a blog post.
A.I. insiders also believe they can fight bias by making their workforces in the U.S. more diverse--always a hurdle for Big Tech. Fei-Fei Li, the Google executive, recently cofounded the nonprofit AI4ALL to promote A.I. technologies and education among girls and women and in minority communities. The group's activities include a summer program in which campers visit top university A.I. departments to develop relationships with mentors and role models. The bottom line, says AI4ALL executive director Tess Posner: "You are going to mitigate risks of bias if you have more diversity."
http://fortunedotcom.files.wordpress.com/2018/06/y.png?w=150&h=15 150w, http://fortunedotcom.files.wordpress.com/2018/06/y.png?w=300&h=29 300w, http://fortunedotcom.files.wordpress.com/2018/06/y.png?w=768&h=75 768w" sizes="(max-width: 1024px) 100vw, 1024px">
YEARS BEFORE this more diverse generation of A.I. researchers reaches the job market, however,big tech companies will have further imbued their products with deep-learning capabilities. And even as top researchers increasingly recognize the technology's flaws--and acknowledge that they can't predict how those flaws will play out--they argue that the potential benefits, social and financial, justify moving forward.
"I think there's a natural optimism about what technology can do," says Candela, the Facebook executive. Almost any digital tech can be abused, he says, but adds, "I wouldn't want to go back to the technology state we had in the 1950s and say, 'No, let's not deploy these things because they can be used wrong.' "
Horvitz, the Microsoft research chief, says he's confident that groups like his Aether team will help companies solve potential bias problems before they cause trouble in public. "I don't think anybody's rushing to ship things that aren't ready to be used," he says. If anything, he adds, he's more concerned about "the ethical implications of not doing something." He invokes the possibility that A.I. could reduce preventable medical error in hospitals. "You're telling me you'd be worried that my system [showed] a little bit of bias once in a while?" Horvitz asks. "What are the ethics of not doing X when you could've solved a problem with X and saved many, many lives?"
The watchdogs' response boils down to: Show us your work. More transparency and openness about the data that goes into A.I.'s black-box systems will help researchers spot bias faster and solve problems more quickly. When an opaque algorithm could determine whether a person can get insurance, or whether that person goes to prison, says Buolamwini, the MIT researcher, "it's really important that we are testing these systems rigorously, that there are some levels of transparency."
Indeed, it's a sign of progress that few people still buy the idea that A.I. will be infallible. In the web's early days, notes Tim Hwang, a former Google public policy executive for A.I. who now directs the Harvard-MIT Ethics and Governance of Artificial Intelligence initiative, technology companies could say they are "just a platform that represents the data." Today, "society is no longer willing to accept that."
This article originally appeared in the July 1, 2018 issue of Fortune.
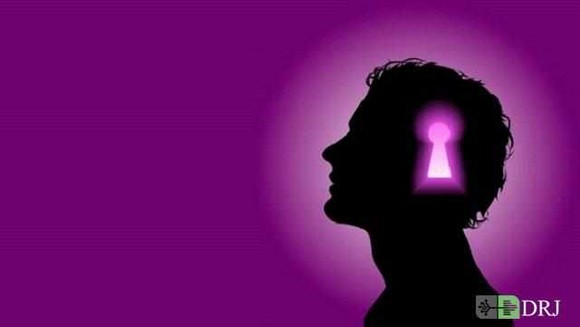
ایده ها برای استارت آپ موجب رونق کسب و کارهای اینترنتی
آینده / استارت آپ
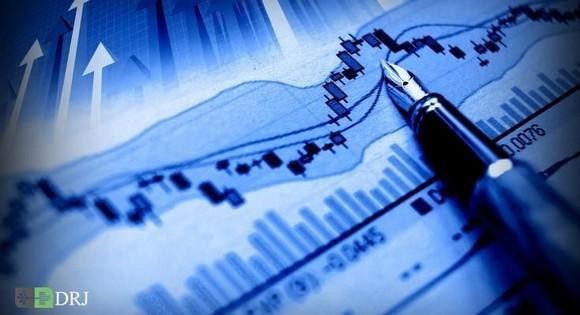
استارتآپها ادبیات بازار سرمایه را بلدند؟
استارت آپ
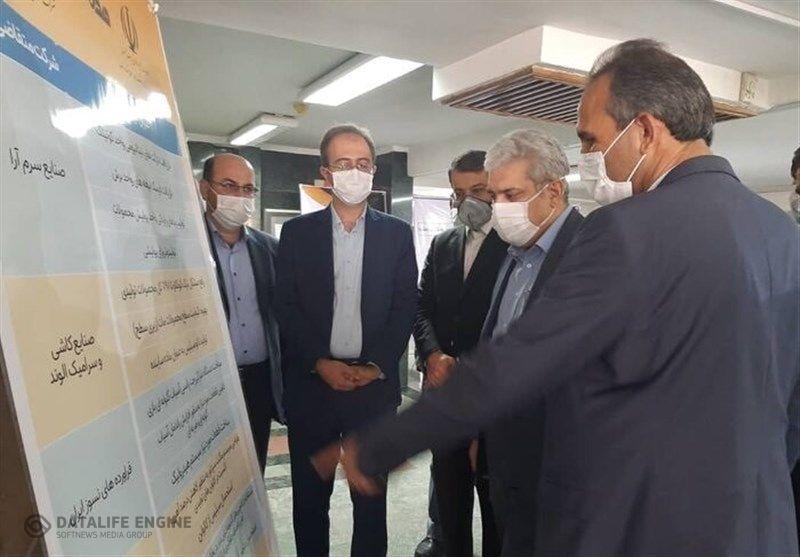
صدور تاییدیه دانش بنیانی شتابدهنده صدر فردا
اخبار / استارت آپ
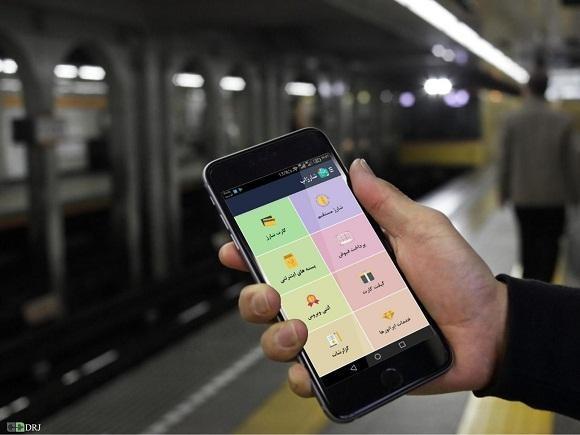
اپلیکیشن شارژاپ
گوناگون / استارت آپ / رپرتاژ آگهی / بازتاب
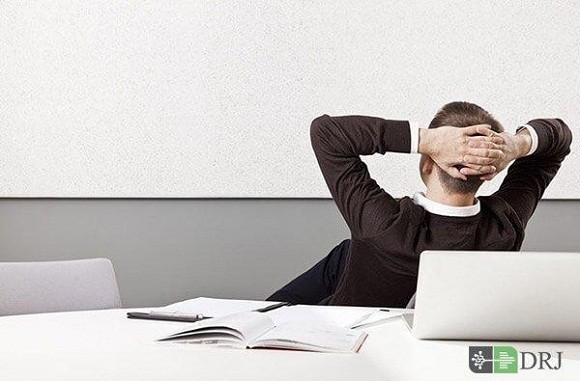
جذابترین ایدههای B2B در سال 2020
استارت آپ
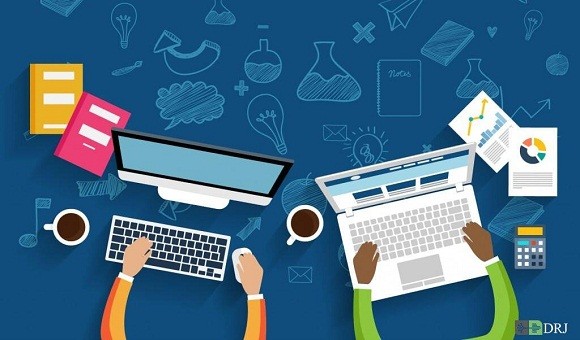
تعریف استارت آپ startup
دانشنامه / استارت آپ / مقاله
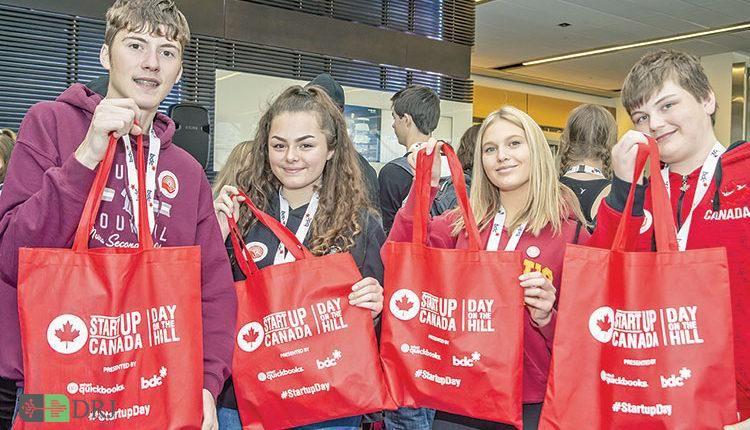
۱۰ استارتاپ که بدون سرمایه به سوددهی رسیدند
استارت آپ
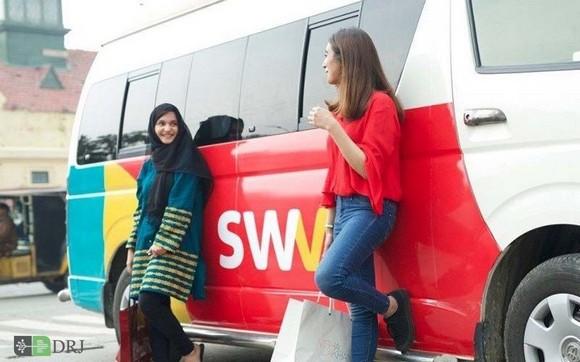
ایده ها و پیشنهاد برای استارت آپ در سال جدید
راهکارها و ترفند ها / استارت آپ
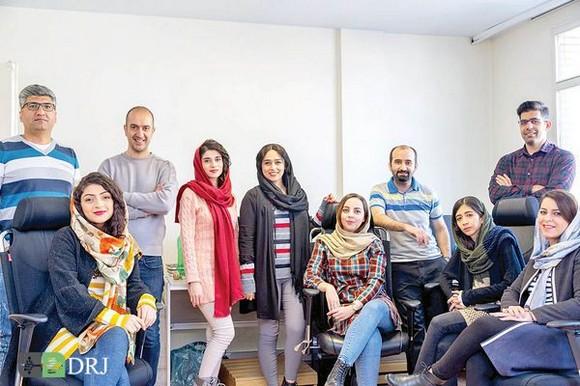
استارتآپ ایرانی؛ مرجع اول زنان افغان
استارت آپ
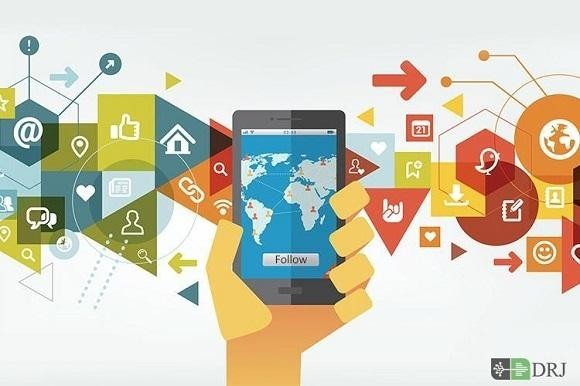
شروع یک کسب و کار نوپا پلتفرمی
استارت آپ
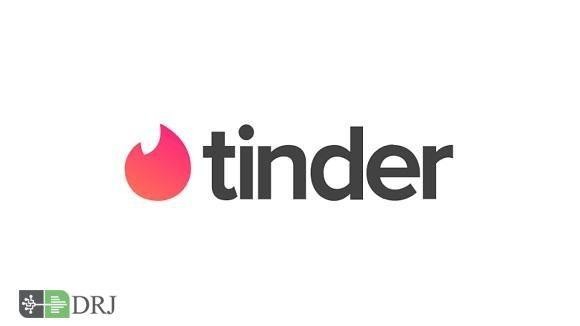
برنامه شبکه اجتماعی تیندر
گوناگون / معرفی وب سایت / استارت آپ
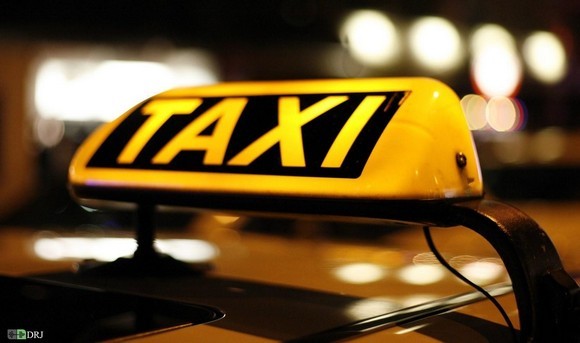
10 استارت آپ برتر تاکسیرانی جهان
استارت آپ
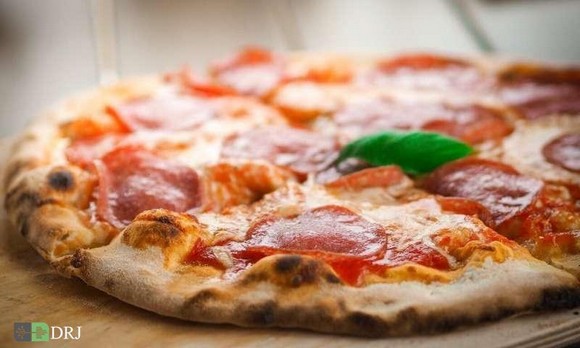
پخت پیتزاهای هیجان انگیز با هوش مصنوعی
آینده / استارت آپ
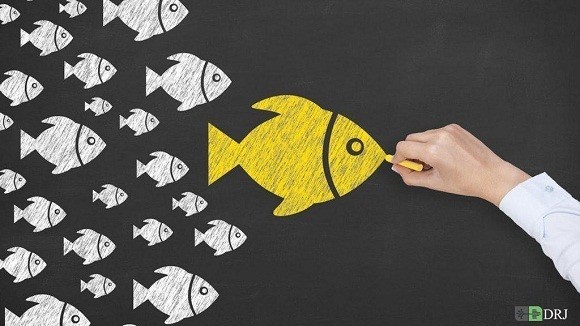
ایده های استارتاپی فراموش شده
دورنما / بازار / استارت آپ
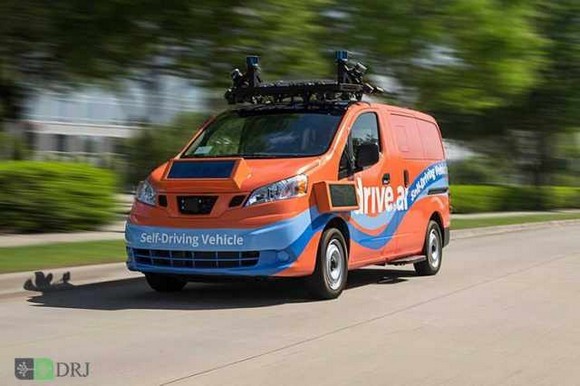
اپل، استارتاپ فناوری خودران Drive.ai را تصاحب کرد
استارت آپ
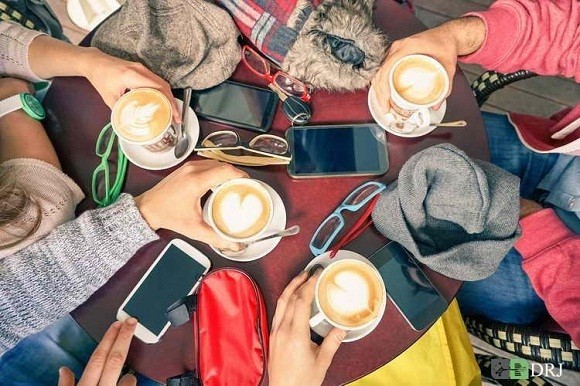
بررسی مهمترین چالشهای تیمهای استارتاپی
استارت آپ
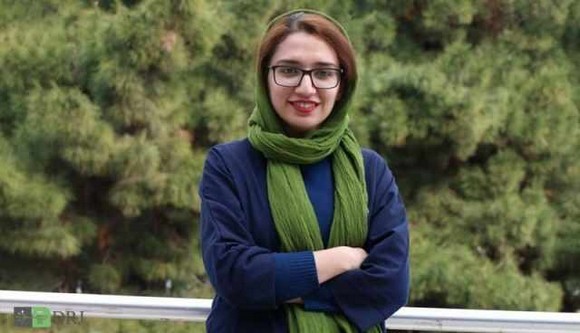
نگرانی کاربران از هزینه تعمیر و تامین قطعات
گفت و گو / بازار / استارت آپ
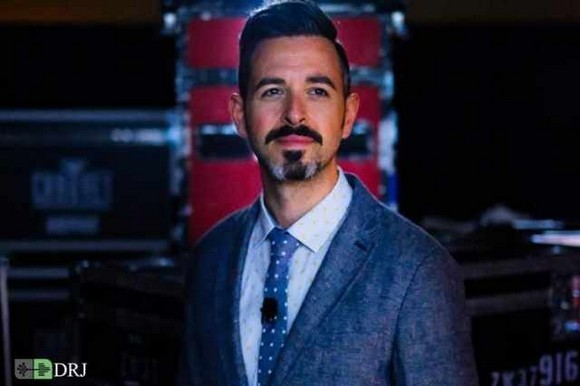
مصاحبه با مدیرعامل و بنیانگذار استارتاپ Moz
گفت و گو / استارت آپ
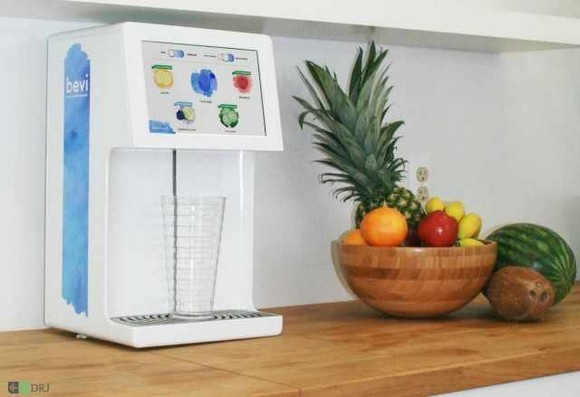
آشنایی با استارت آپ های حوزه مدیریت آب
استارت آپ
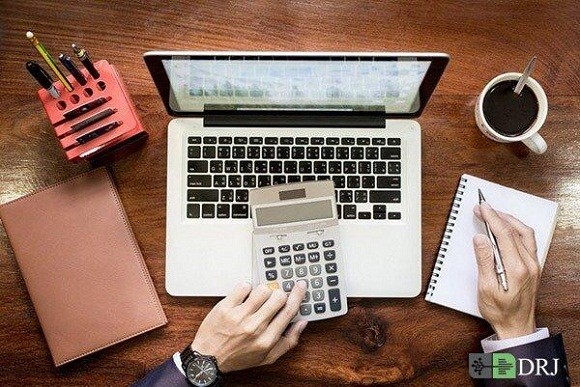
راه اندازی ۷۰ استارت آپ توسط نخبگان ایرانی
استارت آپ
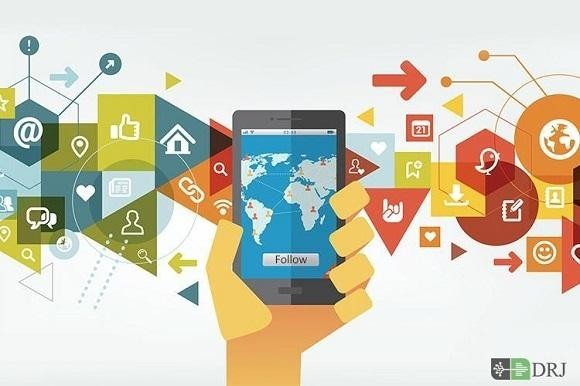
معرفی هشت استارتآپ موفق ایرانی در حوزه فینتک
استارت آپ
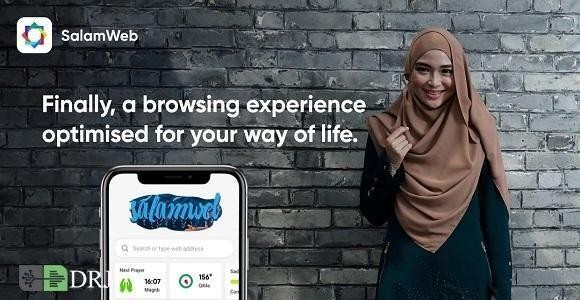
اولین مرورگر شرعی دنیا
استارت آپ
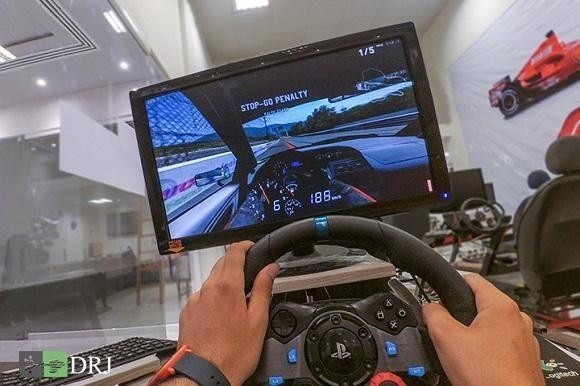
از صفر تا پیست
استارت آپ
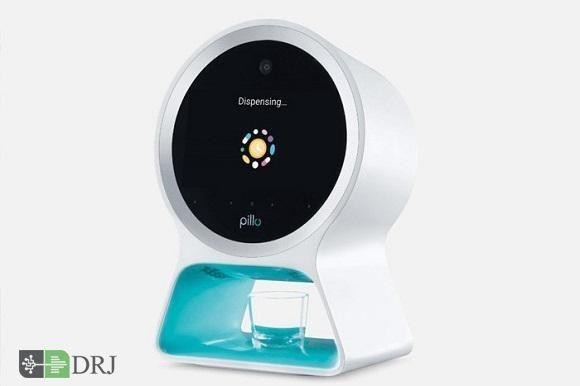
معرفی برترین استارتاپهای CES 2019
اخبار / استارت آپ
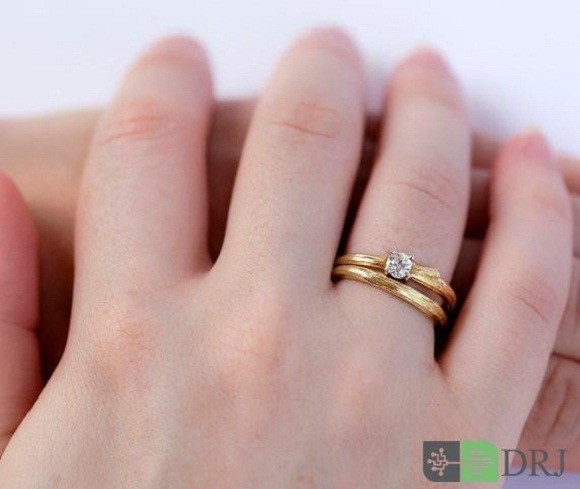
ازدواج با فرد ثروتمند یا خوش اخلاق
سبک زندگی / برترین ها
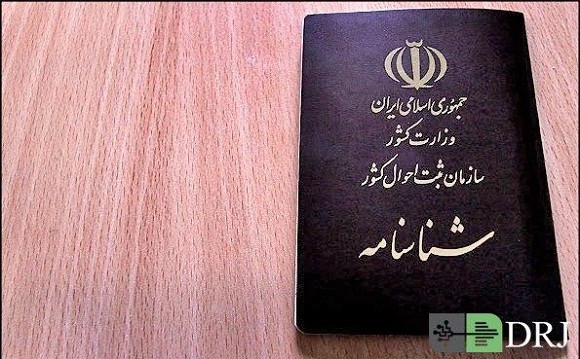
هدف از تشکیل خانواده چیست
سبک زندگی
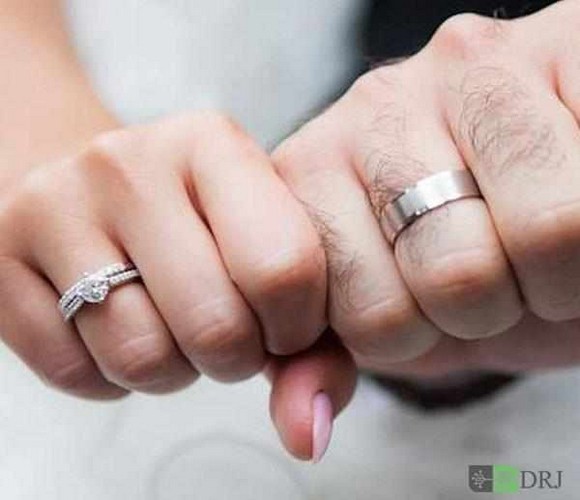
اول عاشق شویم، بعد ازدواج کنیم
سبک زندگی
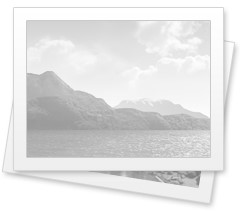
خانواده چیست
سبک زندگی
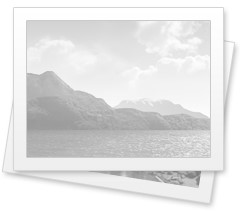
مشاوره خانواده چیست؟
سبک زندگی
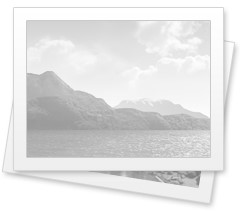
اولویتهای پسانداز خانواده چیست؟
سبک زندگی
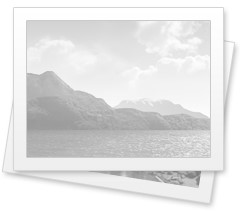
هزینه های خانواده چیست؟
سبک زندگی
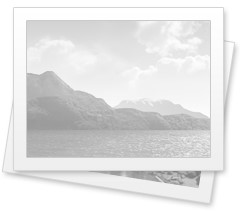
راهکار بیشتر حرف زدن اعضای خانواده چیست؟
سبک زندگی
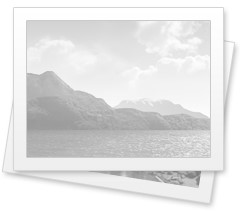
چرخه زندگی و خانواده چیست؟
سبک زندگی
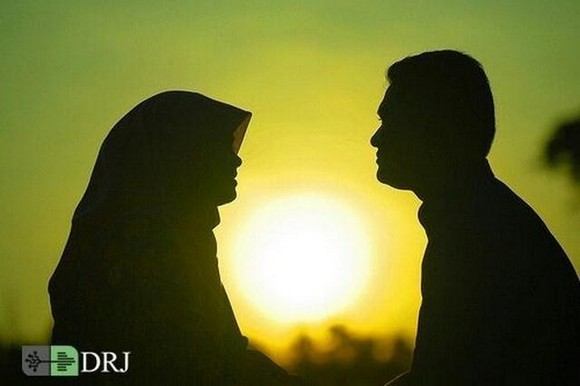
اهداف و اصول تشکیل خانواده
سبک زندگی
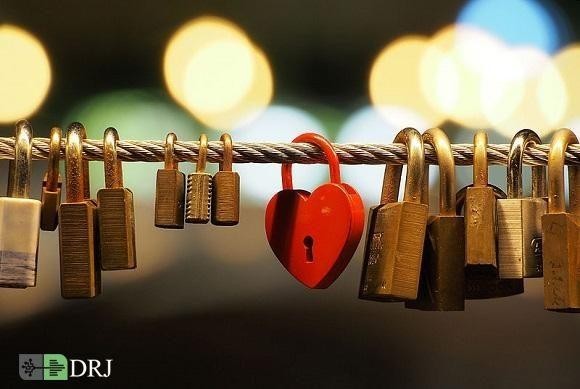
آموزش جنسی نادرست به سبک خانم جلسه ای
سبک زندگی
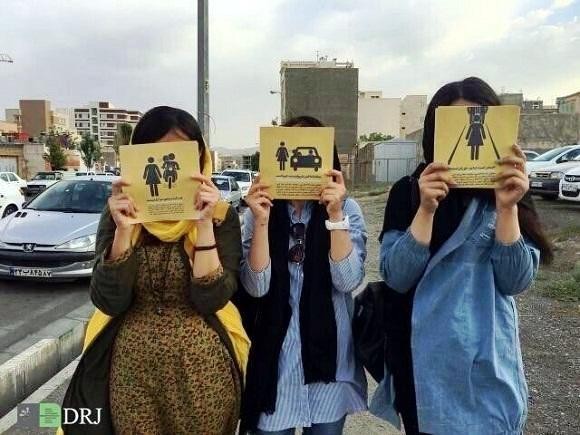
لطفا تماشاچی آزار زنان نباشید!
سبک زندگی
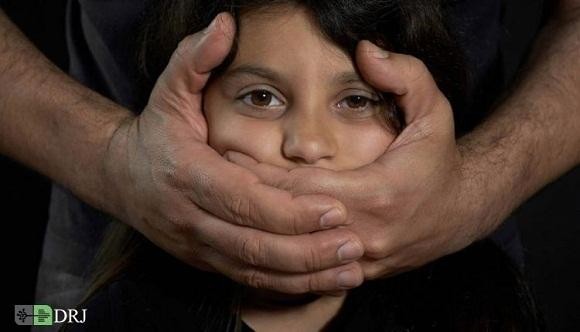
کودک آزاری؛ از نشانهها و دلایل تا درمان
گزارش / سبک زندگی / پرورش کودکان
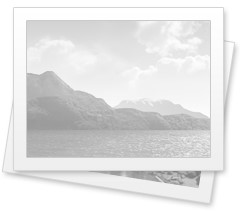
روش های تعیین هدف و مسیر زندگی برای رسیدن به موفقیت
سبک زندگی
مجله اینترنتی دیپروتد نشریه مجازی بر بستر اینترنت به مسائل آموزشی و مقالات پیرامون کسب وکار های نوپا یا استارت آپ ها و سبک زندگی است فعالیت و محتوای مطالب ارائه شده در سایت همه بیشتر در حوزه مدیریت، کارآفرینی ، روانشناسی ،اقتصادی و فناوری اطلاعات است نام اصلی دیپروتد "ریشه های عمیق " با مجوز رسمی از هیات نظارت برمطبوعات مشغول به فعالیت است
ما را در شبکه های اجتماعی دنبال کنید
تمامی حقوق برای سایت فوق محفوط است.
S-TECH: ایرانی توانمند | Powered by: مجله اینترنتی دیپروتد